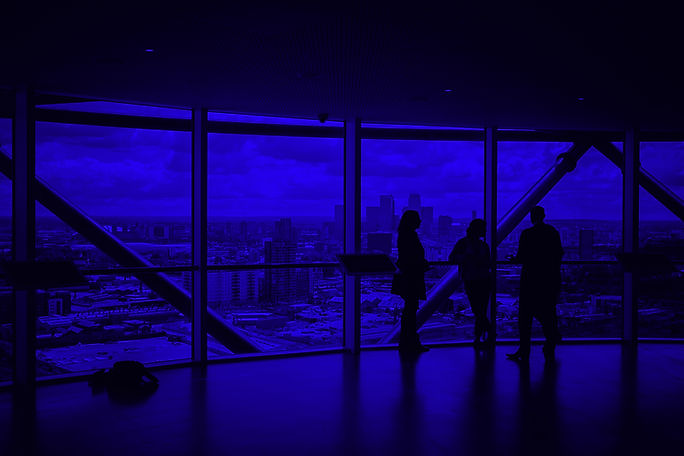
Guest Speakers
Plenary speech 1
The Affordances of Using Learning-Oriented Assessment
as an Assessment Design Framework for Complex Assessments
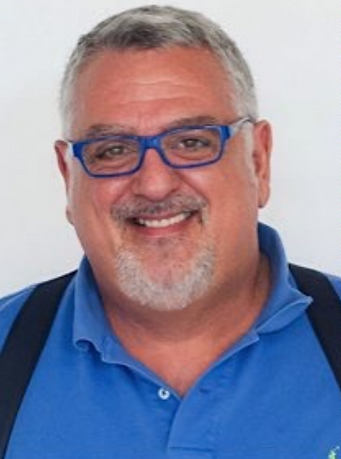
James E. Purpura
Professor of Linguistics and Education in the Applied Linguistics and TESOL Program at Teachers College, Columbia University
James E. Purpura is Professor of Linguistics and Education in the Applied Linguistics and TESOL Program at Teachers College, Columbia University. Jim’s research has focused on grammar and pragmatics assessment, the cognitive dimension of L2 performance, learning-oriented assessment in formal and spontaneous assessment contexts, and scenario-based assessment within and across languages. Jim was President of ILTA, an Expert Member of EALTA, Editor of Language Assessment Quarterly, and a Fulbright Scholar at the University for Foreigners of Siena. Currently, he is Expert Member of ALTE and a long-term member of the US Department of Defense Language Testing Advisory Panel. Jim is currently co-editor of New Perspectives on Language Assessment. He is also Director of the Scenario-Based Assessment Lab at TC and Principal Investigator for a multi-year project designed to Strengthen Integrated Language and Content Instruction in the Algerian Higher Education Context.
Abstract
Over the years several researchers (e.g., Bachman, 1990; Bachman & Palmer, 1996, 2010; Carroll, 1968; Davidson & Lynch, 2002; Lado, 1960) have proposed frameworks for the construction of language assessments. These frameworks have focused on the specification of task characteristics, which serve to control the elicitation of performance consistencies on language tests. Many of the frameworks also align with models L2 proficiency to specify which language use behaviors are likely to be elicited by any one task. In other words, the core focus of assessment is on the proficiency and elicitation (task) dimensions of assessment events.
A further concern for testers, especially in the development and validation of performance assessments (McNamara, 1996), task-based assessments (Norris, 2017), or more recently, scenario-based assessments (Purpura, 2019), relates to the importance of aligning assessments with real world purposes, that is, ensuring the features of test tasks correspond to those of real world tasks, so that performance on test tasks can generalize to performance on tasks in the language use domain (Bachman & Palmer, 1996). In this respect, a third dimension of an assessment event, the contextual dimension, is an essential factor in assessment design frameworks.
While these frameworks are efficient in the design and measurement of relatively simple independent skills tasks situated within the confines of a hermetic task situation capable of targeting limited constructs of interest, and while well-established measurement models exist to evaluate evidence of performance consistencies across test facets, these frameworks are actually ill-equipped to provide a systematic means of designing or analyzing performance in more complex language use situations, where interpretations of success do not depend on the ability to succeed on a single decontextualized, independent skills task, but rather on the ability to succeed across a coherent sequence of interrelated tasks (e.g., collaborative problem-solving). This inadequacy is further highlighted in the assessment of language use that is situated within a sociocultural context, requires the integration of more than linguistic resources (e.g., topical, socio-cognitive, interactional, affective), and might require examinees not only to process but also remember test input, so that they can use this information to communicate in ways that reflect the accumulation of understandings acquired across the language use event.
One solution to addressing these inadequacies is through evidence-centered design (ECD) (Mislevy et al, 2002; Mislevy & Riconscente, 2005), which highlights not only the interplay between the proficiency dimension, elicitation (task) dimension, and contextual dimensions (domain analysis) of an assessment event, but also provides mechanisms for both understanding performance across a sequence of interrelated tasks in a language use situation, and relating design to analysis through evidential reasoning.
It remains unclear, however, how these frameworks might handle the design and analysis of assessments contextualized within language use situations, where instruction, in the form of test input, assistance, or feedback play a role in the event, where learning is expected, and where the assessment event is mediated through social interaction, especially considering how the instructional, socio-cognitive, and social-interactional dimensions of the event can moderate performance. In these assessment contexts, a learning-oriented framework of assessment might help explain the different dimensions of the assessment event and highlight their interplay.
In this talk I will examine the strengths and weaknesses of several assessment frameworks, arguing that they all fail to account for critical dimensions of assessment event when language use is assessed in complex performance assessment situations. I will use an example of complex assessment event to illustrate and describe the different dimensions of the LOA framework along with their synergies. If time, I will also illustrate this with a spontaneous classroom assessment mediated by interaction.
Plenary speech 2
Aligning Large-Scale and Classroom Assessment
Nick Saville
- Director of Research & Thought Leadership at
Cambridge Assessment English
(University of Cambridge)
- Elected Secretary-General of the Association of
Language Testers in Europe(ALTE)
Dr Nick Saville is Director of Research & Thought Leadership at Cambridge Assessment English (University of Cambridge), and is the elected Secretary-General of the Association of Language Testers in Europe (ALTE).
He regularly presents at international conferences and publishes on issues related to language assessment. His research interests include assessment and learning in the digital age; the use of ethical AI; language policy and multilingualism; the CEFR; and Learning Oriented Assessment (LOA). He co-authored a volume on LOA with Dr Neil Jones (SiLT 45, CUP) and recently wrote a chapter on LOA as a way of understanding and using all types of assessment to support language learning (Learning-Oriented Language Assessment, Routledge).
Nick was a founding associate editor of the journal Language Assessment Quarterly and is currently joint editor of the Studies in Language Testing (SiLT, CUP) and editor of the English Profile Studies series (EPS, CUP). He sits on several Cambridge of University Boards, including: the Interdisciplinary Research Centre for Language Sciences; the Institute for Automated Language Teaching and Assessment; and English Language iTutoring (ELiT), providing AI-informed automated systems. He is on the Board of Trustees for The International Research Foundation (TIRF) and was a member of the Advisory Council for the Institute for Ethical AI in Education whose final report was published in March 2021.
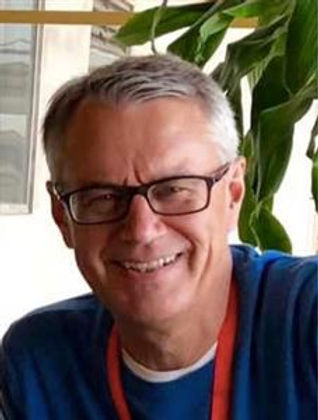
Abstract
The alignment of learning and assessment goals is required to ensure that what is taught is, indeed, what is tested, and that both serve purposes deemed to be of value to society. Alignment is not a simple notion, but is better understood as a ‘complex, non-linear, interacting system’ (Daugherty et al 2008:253), within an Ecosystem of Learning. I will explore different interpretations of goals and alignment, and their practical implications.
Plenary speech 3
Making Assessment Work in the Classroom
David Booth
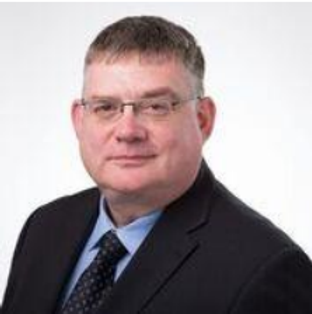
The Director of Test Development for
Pearson English Assessment.
The Director of Test Development for
Pearson English Assessment. He is responsible for the
development of specific Pearson tests ensuring that all test
development processes are executed to the highest
standards and that test material is of the highest quality and
fit for purpose. David works closely with other staff at
Pearson to develop assessment and learning solutions to
meet specific customer requirements.
David’s main expertise is in the development and revision of
tests and he has given presentations at major conferences on
this theme. David has also contributed articles on specific test
development projects in published research notes. David’s
other interests include corpus linguistics and assessment for specific purposes.
Before joining Pearson, David worked for 10 years at Cambridge ESOL, a part of Cambridge
Assessment. David also has extensive academic management, teaching and teacher training
experience working for the British Council in South Korea, Hong Kong and Malaysia
Abstract
The classroom is a busy place with teachers presenting and practicing language points and skills, encouraging learners to communicate effectively in groups, whilst paying attention to the social context of language use. In addition, teachers offer skills practice and helpful learning strategies often in relation to the specific goals of the learner, for future study or developing language skills appropriate for the workplace. Similarly, teachers are involved in evaluating learners; sometimes just to give feedback to support learning but also for end of term or end of year evaluations which can have an impact on the learners’ progress, motivation and life chances. This testing activity is often set against the background of high stakes international tests of English such as PTE Academic or IELTS.
Set in the broader context of modern language assessment practices, this paper will look at specific classroom tools which help teachers evaluate students learning and gives comprehensive feedback referencing specific learning objectives and course material designed to have an immediate impact on the learner. The paper will evaluate the use of automated scoring methods based on AI (artificial intelligence) technologies for productive language skills, such as those used in Pearson Benchmark tests and PTE Academic, contrasting them with the traditional models of speaking and writing assessment.
The paper will also look at how using test items which target integrated skills taps into a wider range of language ability traits thereby challenging the construct under-representation of current approaches to testing. This approach adds significant detail and precision essential to high stakes tests such as PTE Academic but also fundamental to tests such as Pearson Benchmark where detailed feedback is needed. These tools and approaches help build a much more comprehensive picture of language learning for both the learner and the teacher and for younger learners, the parent, identifying where the learner could work most actively to improve their language proficiency. The assessment tools are also used in conjunction with certificated tests to reward learners throughout their lifetime of language learning.
Plenary speech 4
The State-of-the-Art in Applications of Artificial Intelligence (AI) for English Language Assessments
Alistair Van Moere
- Chief Product Officer at MetaMetrics
- Research Professor at University of
Carolina at Chapel Hill.
Chief Product Officer at MetaMetrics and Research Professor at University of Carolina at Chapel Hill. He drives research in assessment and AI technologies, and helps organizations make sense of measurement and test scores. Alistair was previously president of Pearson Knowledge Technologies, where he managed artificial intelligence scoring services for speaking and writing for Pearson’s high-stakes tests. He also oversaw development and delivery of large-scale assessments for millions of learners. Alistair has worked as a teacher, examiner, director of studies, university lecturer and test developer, in the U.S., U.K., Japan and Thailand. He has an MA in English Language Teaching, Ph.D. in Language Testing, and an MBA. He is frequently invited to speak at conferences and has authored over 20 research publications with a focus on educational technology and language assessments.

Abstract
This paper introduces current topics and research in Artificial Intelligence (AI) techniques for the scoring of speaking and writing in English language assessments. The paper is organized around several main themes which are central to the use of AI.
First, the importance of “training” data required to develop AI scoring engines is discussed. There is a growing awareness of the importance of representative training data, and how bias in training data results in bias outcomes. We will investigate examples where sparse data or data unrepresentative of the target test audience may lead to potentially damaging results, for example, uneven performance in the accuracy of speech recognition across different populations.
Next, the features that comprise automated scoring models are analyzed, for both writing and speaking. We will review the commonly-used features (or variables) in scoring models and compare them with typical descriptors in rating criteria to evaluate their fit with the intended construct. Also examined are the differences between “surface features” such as punctuation or vocabulary frequency which are readily understandable - and often gameable - versus harder-to-gauge techniques from the field of natural language processing. The visible, verifiable approach of feature analysis and modeling (“white box”) is contrasted with deep learning approaches where the inner workings of the algorithms are difficult for humans to trace (so-called “black box”).
Last, validity is discussed with reference to the kind of additional analyses that we should expect to see in AI-scored assessments. While the gathering of validity evidence is an ongoing process, there are certain crucial data which allow stakeholders to properly evaluate a test that should be reported from the outset of test publication, particularly with regard to the performance of machine scoring and interpretation of AI-based scores.
Throughout this presentation, the benefits and limitations of certain approaches to AI scoring are described, with a view to educating the audience so that they are better able to understand and evaluate AI-scored assessments.